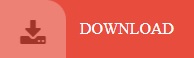
Due to the immature development of the anatomical and physiological structures of preschool children, and the common thymus shadow and transverse heart in infants and young children, children's chest x-ray images generally have image problems such as the unclear boundary of lung field and severe inter-organization interference, which brings great difficulties to lung tissue segmentation ( 8, 9). But the diagnosis of these diseases relies on the precise segmentation of the lungs in children's chest x-rays ( 9). Lung diseases are the leading cause of death among children in many countries, mainly including bronchiolitis, bronchitis, bronchopneumonia, interstitial pneumonia, lobar pneumonia and pneumothorax. These computational methodologies can assist physicians with making a timely, accurate medical diagnosis to improve the quality of care and outcome for patients. Correct identification of lung fields enables further computational analysis of these anatomical regions, such as extraction of clinically relevant features to train a machine learning algorithm for the detection of disease and abnormalities. Lung segmentation is the process of accurately identifying lung field regions and boundaries from surrounding thoracic tissue and is therefore an important first step in lung image analysis for many clinical decision support systems, such as detection and diagnosis of Covid-19 ( 3– 5), classification of pneumonia ( 6), detectionof tuberculosis ( 7), etc. Therefore, chest x-ray has become one of the most commonly used medical diagnostic methods to detect children's lung diseases. Among routine imaging examinations, x-ray examination, especially chest film examination, occupies a large proportion in children's photography due to its advantages of low dose radiation, convenient examination and low cost, accounting for more than 40% of all imaging diagnoses, of which infant chest photography accounts for more than 60% of the whole pediatric x-ray examination ( 1, 2). With the development of modern medicine, the importance of medical imaging has become increasingly prominent. Results: Applied on the test set containing multi-center data, our model achieved a Dice score of 0.9822.Ĭonclusions: This novel lung segmentation method proposed in this work based on TransResUNet is better than other existing medical image segmentation networks. The former can provide a self-attention module improving the feature learning ability of the model, while the latter can avoid the problem of network degradation. Methods: The novelty of the proposed method is the combination of merits of TransUNet and ResUNet. Objective: In this study, we propose a method based on deep learning to improve the lung segmentation quality and accuracy of children's multi-center CXR images. Lung field segmentation in digital CXR images is a key section of many computer-aided diagnosis systems. 9Polytechnic Institute, Zhejiang University, Hangzhou, Chinaīackground: Chest x-ray (CXR) is widely applied for the detection and diagnosis of children's lung diseases.8Department of Information Standardization Research,National Institute of Hospital Administration, NHC, Beijing, China.

7Department of Radiology, Children’s Hospital, Zhejiang University School of Medicine, Hangzhou, China.6Department of Scientific Research, Avaintec Oy Company, Helsinki, Finland.5Department of Neurology, The Warren Alpert Medical School of Brown University, Providence, RI, United States.4Department of Scientific Research, Huiying Medical Technology (Beijing) Co., Ltd, Beijing, China.3Medicine Engineering and Information Research Institute for Children's Health, National Clinical Research Center for Child Health, Hangzhou, China.2Medicine Engineering and Information Research Institute for Children's Health, Sino-Finland Joint AI Laboratory for Child Health of Zhejiang Province, Hangzhou, China.

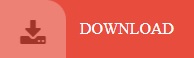